Research
1. Molecular alterations in prostate cancer
We study molecular alterations that occur during prostate cancer development and treatment resistance. With collaborative large-scale sequencing and proteomics efforts we have screened prostate cancer patient tumors for novel, previously unrecognized molecular alterations. We study them further to understand their significance for cancer formation and treatment responses, and search for novel ways for therapeutic targeting.
Hormone-dependent cancers are driven by steroid hormone receptors, such as androgen and estrogen receptors, which function as transcription factors that regulate expression of other genes. Although many current cancer drugs are targeting the activities of these receptors, treatment resistance is common and better treatments are needed.
Recent publications:
Latonen et al. 2018 Nature Communications: Integrative proteomics in prostate cancer uncovers robustness against genomic and transcriptomic aberrations during disease progression.
Scaravilli et al. 2020 Current Opinion in Endocrine and Metabolic Research: Integrative proteomics of prostate cancer.
Uusi-Mäkelä et al. 2020 bioRxiv 2020.09.08.287268: Chromatin accessibility analysis uncovers regulatory element landscape in prostate cancer progression.
Scaravilli et al. 2021 Front. Cell Dev. Biol: Androgen-Driven Fusion Genes and Chimeric Transcripts in Prostate Cancer.
Eerola et al. 2021 Cancer Medicine: Expression and ERG-regulation of PIM kinases in prostate cancer.
Sadeesh et al. 2021 Cancers: Proteomic Landscape of Prostate Cancer: The View Provided by Quantitative Proteomics, Integrative Analyses, and Protein Interactomes.
Kukkonen et al. 2022 Endocrine-Related Cancer: Nonmalignant AR-positive prostate epithelial cells and cancer cells respond differently to androgen.
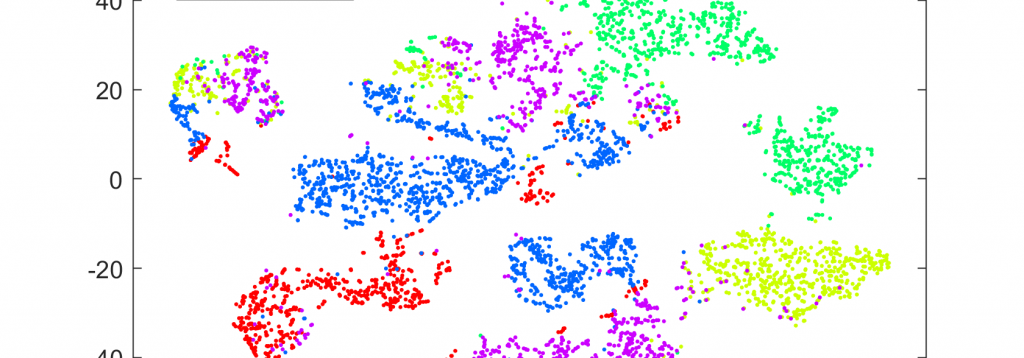
2. Functions of RNA and RNA-binding proteins in cancer
We are interested in alterations of RNA expression and regulation in cancer. We study the functional role of several non-coding RNAs with aberrated expression in prostate cancer, including microRNAs and lncRNAs. We especially focus on RNA-binding proteins and their role in regulating mRNA and other species of RNA in cancer cells. While many of these RNA-binding proteins have a recognized role in several other types of diseases, such as neurodegenerative aggregation diseases, their roles in cancer are mostly unexplored and they pose a potential group of novel cancer drug targets.
Recent publications:
Latonen et al. 2017 Am J Pathol: In Vivo Expression of miR-32 Induces Proliferation in Prostate Epithelium.
Kohvakka et al. 2020 Oncogene: AR and ERG drive the expression of prostate cancer specific long noncoding RNAs.
Scaravilli et al. 2022 Oncogenesis: miR-32 promotes MYC-driven prostate cancer.
Sattari et al. 2023 Endocr Relat Cancer: Identification of long noncoding RNAs with aberrant expression in prostate cancer metastases.
Aikio et al. 2023 Cancer Reports: Complementary analysis of proteome-wide proteomics reveals changes in RNA binding protein-profiles during prostate cancer progression.
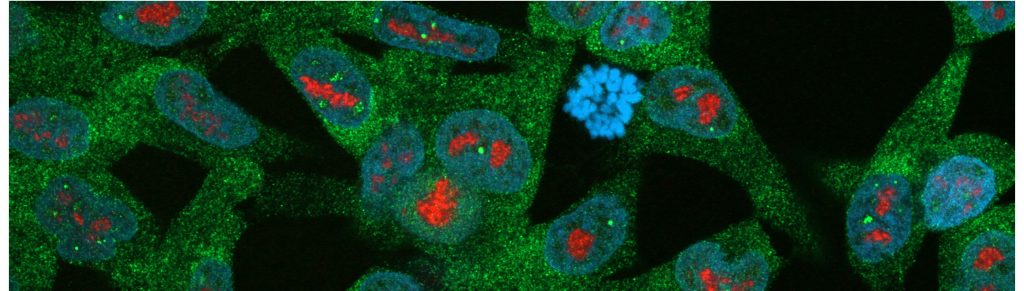
3. The stress-responsive nuclear organelles
Several types of stress induce accumulation of proteins and RNA in cells. These stress responses are well studied in the cytoplasm, but the events in the nucleus are less well known. We have shown that the nucleolus is a site of accumulation and aggregation during transient stress, serving most likely as a safe harbor for unwanted molecular entities. Now we work to understand the role of the nucleolus and other nuclear bodies in formation of cancer and drug resistance.
References:
Batnasan et al. 2024 Cancer Cell International: Platinum-based drugs induce phenotypic alterations in nucleoli and Cajal bodies in prostate cancer cells.
Batnasan E. 2022 Results and Problems in Cell Differentiation: Nuclear Organization in Response to Stress: A Special Focus on Nucleoli.
Latonen L. 2019 Frontiers in Cellular Neuroscience: Phase-to-Phase With Nucleoli – Stress Responses, Protein Aggregation and Novel Roles of RNA.
Latonen et al. 2011 Oncogene: Proteasome inhibitors induce nucleolar aggregation of proteasome target proteins and polyadenylated RNA by altering ubiquitin availability.
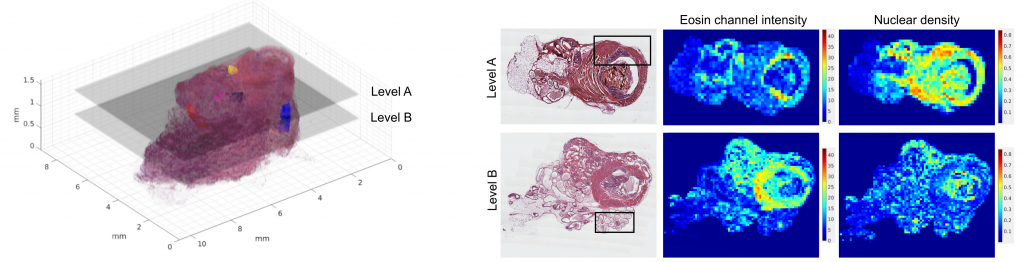
4. Quantitative tissue analysis of cancer
We query cancer growth patterns in tissue and combine this to molecular information in order to better understand how cancer develops and grows. We search for better ways to image, visualize, and quantitatively analyse histology, utilizing multiple and novel imaging modalities as well as digital pathology, machine learning, and AI tools.
We belong to the international ERA PerMed-funded ABCAP consortium, aim of which is to develop and validate novel state-of-the-art deep learning-based computer models for improved routine histopathology classification and for refined patient stratification in breast cancer. Within the consortium, we test the utility of different microscopic and spectroscopic methods in AI-based cancer tissue identification and grading.
Recent publications:
Ehteshami Bejnordi et al. 2017 JAMA: Diagnostic Assessment of Deep Learning Algorithms for Detection of Lymph Node Metastases in Women With Breast Cancer.
Kartasalo et al. 2018 Bioinformatics: Comparative analysis of tissue reconstruction algorithms for 3D histology.
Liimatainen et al. 2021 Biomolecules: Convolutional Neural Network-Based Artificial Intelligence for Classification of Protein Localization Patterns.
Liimatainen et al. 2021 BMC Cancer: Virtual reality for 3D histology: multi-scale visualization of organs with interactive feature exploration.
Latonen and Ruusuvuori, 2021 European Journal of Cancer: Building a central repository landmarks a new era for AI-assisted digital pathology development in Europe.
Ruusuvuori et al. 2022 Heliyon: Spatial analysis of histology in 3D: quantification and visualization of organ and tumor level tissue environment.
Koivukoski et al. 2023 Laboratory Investigation: Unstained Tissue Imaging and Virtual Hematoxylin and Eosin Staining of Histologic Whole Slide Images.
Khan et al. 2023 Patterns: The effect of neural network architecture on virtual H&E staining: Systematic assessment of histological feasibility.
Ruusuvuori et al. 2023 Cancer Cell: Deep learning transforms colorectal cancer biomarker prediction from histopathology images.
Latonen et al. 2024 Trends in Biotechnology: Virtual staining for histology by deep learning.
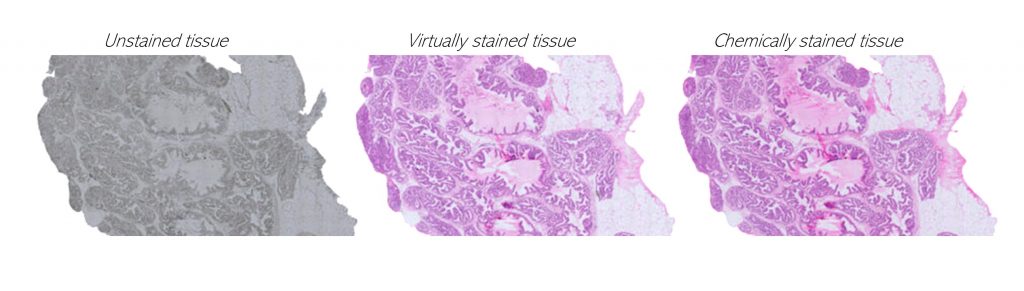