Objective
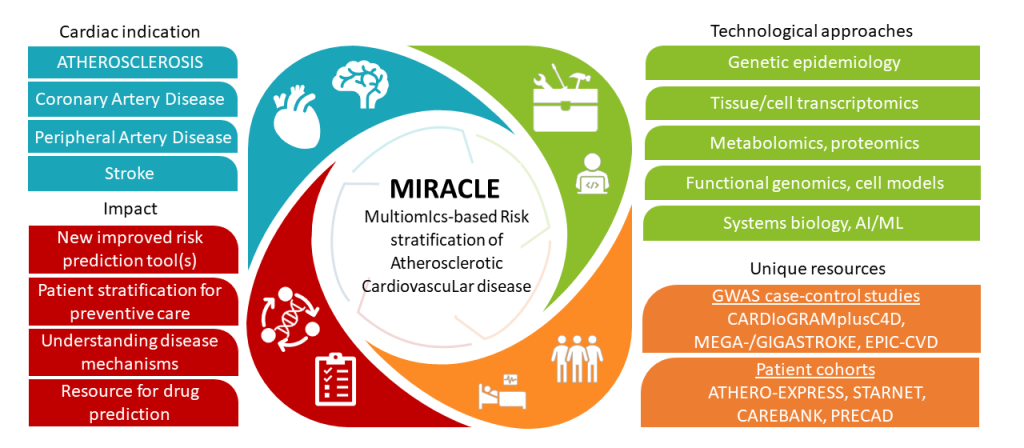
Atherosclerotic cardiovascular disease (ASCVD) is the leading cause of mortality worldwide. Aside from asymptomatic manifestations, the first sign of clinically significant ASCVD is often a severe clinical event, such as stroke or myocardial infarction (MI). Thus, identifying individuals at high risk is crucial in preventing the fatal consequences of ASCVD.
Current risk prediction models based on traditional risk factors, such as SCORE2, have limitations since they do not encompass all mechanisms and intermediary phenotypes leading to ASCVD. Particularly, current risk models fail to consider the disturbance of gene regulatory networks (GRNs) caused by genetic risk factors and diverse longitudinal exposures accumulating during a person’s lifetime. Furthermore the current models predict the combined risk of CAD, PAD and ischemic stroke despite mounting evidence of the heterogeneity of the underlying disease mechanisms.
To capture the missing aspects of current ASCVD risk scores, MIRACLE project brings together unique data resources and expertise to provide novel multiomics based prediction models of ASCVD. We aim to
- Integrate the globally largest CAD, PAD, and stroke GWAS information to identify genetic loci that differ between or are shared by these diseases and their subtypes
- Identify sex-specific subtypes of ASCVD patients using transcriptomic phenotyping of plaques and circulating biomarkers
- Generate functionally informed polygenic risk scores by combining experimental fine-mapping and gene prioritization approaches with integrative GRN and deep learning modelling.
- Derive novel risk prediction models incorporating polygenic risk and circulating biomarkers. Providing a new gold standard for prediction models to accurately risk stratify stroke and MI represents a technological breakthrough allowing for earlier diagnoses and treatments of ASCVD.
