AI4Sleep
New Enhanced and Individualized Diagnostic Paradigm for Obstructive Sleep Apnea
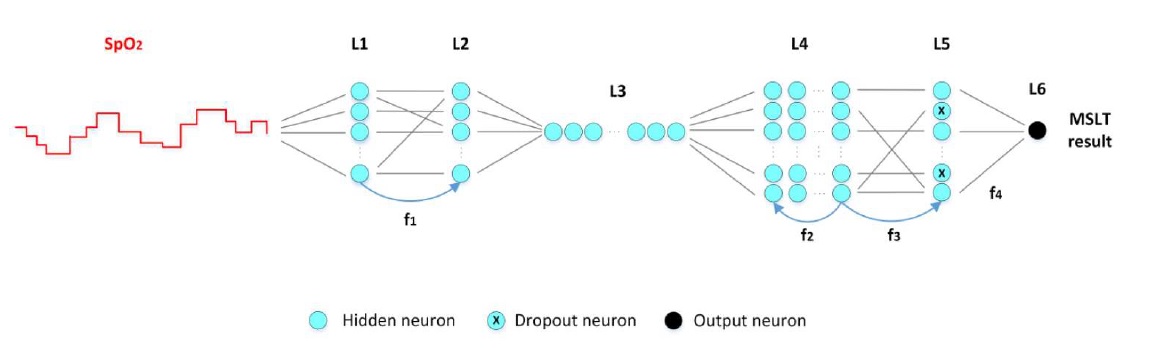
Summary: Obstructive sleep apnea (OSA) affects 9-27% of the middle-aged population, increases risk for cardiovascular morbidities, and has a major socioeconomic impact. Currently, sleep apnea is diagnosed based on daytime sleepiness and apnea-hypopnea index (AHI), which is simply an average number of obstructive events per hour of sleep. This simplistic diagnostic has several major shortcomings significantly limiting the estimation of disease severity. The current diagnosis of sleep apnea is time-consuming and expensive due to manual detection of every single apnea and hypopnea event. Thus, there is a critical and urgent need for methods supporting automatic assessment of OSA severity, making sleep studies more readily available with lower costs. Artificial intelligence algorithms could provide a solution for automated diagnosis of sleep apnea and prediction of the progression of the disease and risk of related co-morbidities, such as stroke and heart failure.
In this project, we aim to revolutionize the diagnosis of sleep apnea by introducing state-of-the-art artificial intelligence techniques: (a) for the automatic diagnosis and assessment of sleep apnea severity; and (b) to predict the progression of the disease and (c) the risk of related co-morbidities, such as stroke and heart failure. In addition, to enhance understanding on the most important aspects needed to be considered in diagnostics, the aim is to introduce also completely new parameters for estimation of OSA severity.
As an outcome, this research could result in innovations that will provide more effective, efficient and personalised pathways for the diagnosis, severity assessment and management of sleep apnea, reducing significantly its socioeconomic impact and associated co-morbidities on the healthcare systems. This project contributes extensively to artificial intelligence-based risk stratification for sleep apnea to prioritize treatment for those patient phenotypes with the highest risk for the related severe health consequences. On the other hand, identifying those patients who will gain only limited benefit from treatments is beneficial for both the individual (no need of unnecessary intrusive treatments) and the community (no need to fund ineffective treatments).
Research period: 1.9.2019-31.8.2022
Funding: Academy of Finland (Call: Postdoctoral Researcher)