Shekhar Singh: How to Analyze Different Samples and Sample Sizes: A Researcher’s Guide
Ever wondered why some research findings seem rock-solid while others feel shaky? It all boils down to how samples are selected and analyzed.
Whether you’re comparing groups, working with different sample sizes, or just trying to make sense of your data, knowing the right statistical approach makes all the difference.
Let’s dive into the fascinating world of sample analysis—with no boring stats lectures.
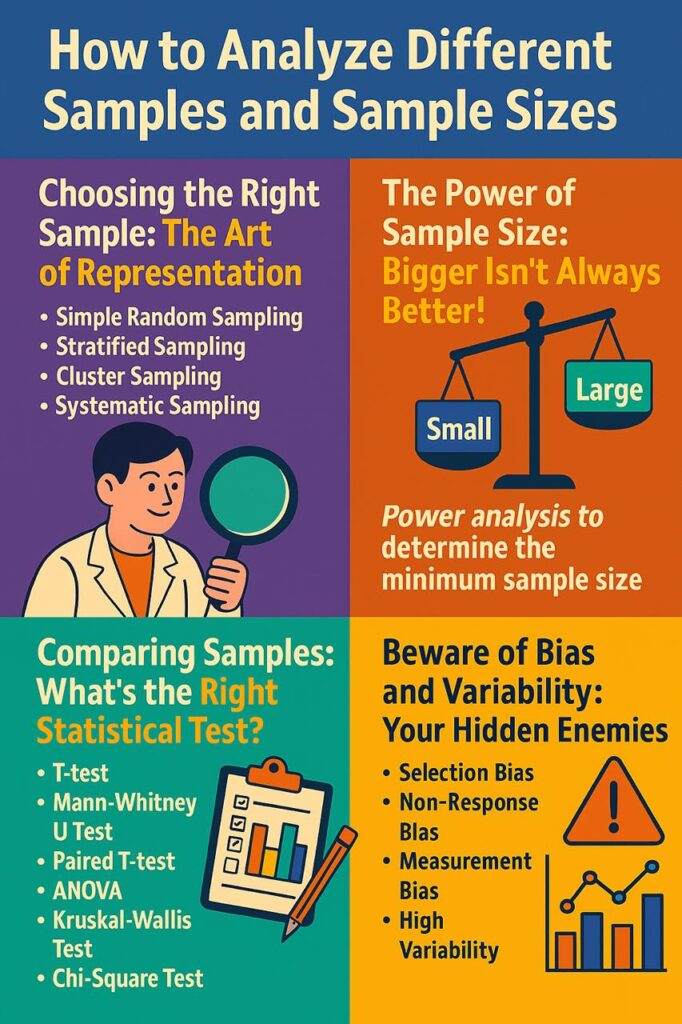
Choosing the Right Sample: The Art of Representation
Your sample is the heart of your study. Pick the wrong one, and your entire research could be misleading! So, how do you ensure your sample represents the population you’re studying?
Here are some common sampling methods—think of them as different strategies for picking the best slice of the pie:
- Simple Random Sampling: Everyone in the population has an equal chance of being selected. It’s the fairest method—like drawing names from a hat!
- Stratified Sampling: When you want balanced representation, divide your population into subgroups (strata) and sample from each. Example: If 60% of your population is female, 40% male, your sample should reflect that.
- Cluster Sampling: Instead of picking individuals, you randomly select entire groups (e.g., classrooms in a school). Great for large populations, but clusters might not always be diverse.
- Systematic Sampling: Pick every nth person from a list—simple, structured, and often more practical than purely random selection.
- Convenience Sampling: The easiest but riskiest method—choosing participants who are readily available. Be warned: this can lead to biased results!
The takeaway?
Your sampling method should match your research goals. If your sample is biased, your results will be, too. So, choose wisely!
The Power of Sample Size: Bigger Isn’t Always Better!
Picture this: You survey 10 people about their coffee habits. Five love espresso, five hate it. Does this mean half the world dislikes espresso? Nope. Your sample is too small!
Sample size matters, but bigger isn’t always better. Let’s break it down:
Small Sample Sizes
✅ Quick and cost-effective.
❌ High chance of random variation skewing results.
❌ More likely to make ‘Type I errors’ (false positives) and ‘Type II errors’ (false negatives).
Large Sample Sizes
✅ More accurate and reliable.
✅ Reduces random variation.
❌ More expensive and time-consuming.
❌ After a certain point, increasing sample size doesn’t add much value—it just eats up resources.
So, what’s the sweet spot?
Use power analysis to determine the minimum sample size needed for meaningful results. This ensures you have enough data to detect real effects without wasting time or money.
Comparing Samples: What’s the Right Statistical Test?
Okay, so you’ve collected your data. Now what? If you compare different samples, you need the right statistical test.
Here’s a quick cheat sheet:
Comparing Two Independent Groups?
- T-test: Great for comparing two group means (e.g., test scores of males vs. females).
- Mann-Whitney U Test: A non-parametric alternative for non-normally distributed data.
Comparing the Same Group Over Time?
- Paired T-test: Use when measuring the same people before and after an event (e.g., weight loss before and after a diet).
Comparing Multiple Groups?
- ANOVA: Compares means across three or more groups (e.g., effectiveness of three teaching methods).
- Kruskal-Wallis Test: The non-parametric version when data isn’t normally distributed.
Analyzing Categorical Data?
- Chi-Square Test: Checks if two categorical variables are related (e.g., gender and voting preference).
- Fisher’s Exact Test: Use when sample sizes are small.
Pro Tip:
Always check for normality before choosing your test! If your data isn’t normally distributed, stick with non-parametric tests.
Final Thoughts: Research Like a Pro!
Analyzing different samples and sample sizes isn’t just about crunching numbers—it’s about making sure your research tells the truth.
Golden rules to remember:
✅ Choose a sampling method that fits your research.
✅ Ensure your sample is large enough, but don’t overdo it.
✅ Pick the right statistical test for comparing samples.
✅ Always check for bias and variability before trusting your results.
Master these, and you’re well on your way to conducting rock-solid research that stands up to scrutiny. Happy analyzing!
Shekhar Singh works as a doctoral researcher in the Neuro-Innovation PhD Programme. His research focuses on epilepsy in patients with CSTB mutation. Read more about Shekhar’s journey here.