Biomedical EIT
Biomedical EIT – Classification of stroke
Stroke is among the leading causes of death. The socioeconomical effects of the disease are aggravated by the fact that survivors often suffer from severe brain damage, and require permanent institutional care. Strokes can be classified into two major categories: ischemic and hemorrhagic. About 80% of strokes are ischemic, caused by a blockage in a vessel (or artery). Rest of the strokes are hemorrhagic. At present, early diagnosis and treatment of stroke has a major problem: For all the differences between ischemic and hemorrhagic strokes, from onset to treatment, they are alike in symptoms. This is unfortunate, since treating a patient suffering from a hemorrhagic stroke with blood-thinning and clot dissolving drugs can prove fatal.
The most important tools for classifying a stroke are Magnetic Resonance Imaging (MRI) and computerized X-ray tomography (CT). Currently we lack methods to separate the stroke types in the acute phase without these imaging devices. Moreover, as CT and MR imaging devices are located at large hospitals, crucial time may be lost due to slow diagnosis; every hour in which a large ischemic stroke is untreated, the brain loses as many neuron as it does in several years of normal aging. Worse still, there is a four and a half hour window in which clot dissolving drugs have shown to be effective, and many patients just don’t make it in time. The situation would be significantly improved if there was a portable stroke classifier that could be used in the ambulance for the classification of the stroke.
Our aim is to improve the classification of strokes using Electrical Impedance Tomography (EIT), where the data is collected by feeding harmless and painless electric currents into the body through electrodes and measuring the resulting voltages at the electrodes. A 3D image of the electrical conductivity of the head is computed from the data using a reconstruction algorithm. The resulting image can then be used for the classification.
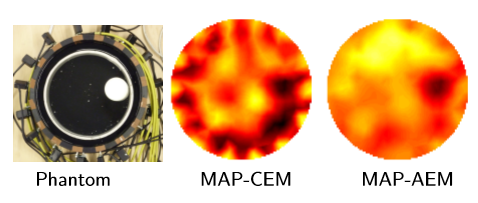
Figure: Simplified example of EIT imaging through poorly conducting (skull) layer. Middle: Reconstruction using conventional approach. Right: Reconstruction where the uncertainty related to the shielding layer has been marginalized using the approximation error approach.
Contact
Past and present collaborators
- Professor Samuli Siltanen, University of Helsinki
- Professor Jari Hyttinen, Tampere University of Technology
- Professor Simon Arridge, University College London