Remote sensing of forest
Remote sensing of forest
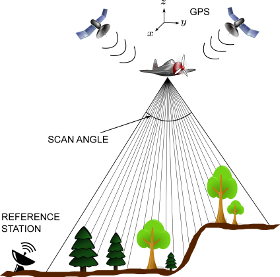
Global and local environmental changes are modifying forest ecosystems rapidly. The consequences of ecosystem changes on biodiversity, functioning of forests, and provision of ecosystem services are largely unclear. The analysis of ecosystem changes in different spatial scales is scientifically challenging. Reliable data of forest characteristics is a fundamental requirement for analysing environmental changes and their impacts. During the last years airborne laser scanning (ALS) has evolved to most accurate source for remote sensing data in forest environment applications. ALS is a remote-sensing technique that provides with three-dimensional (3D) high-precision measurements of targets in the form of a point cloud, based on laser-ranging measurements. The principle of ALS is illustrated in the figure on the right hand side. We develop Bayesian inversion methods for ALS based remote sensing of forest.
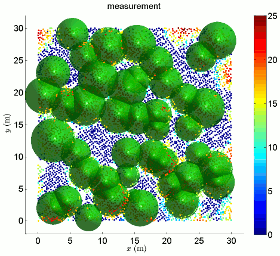
Both area-based and individual tree detection -based approaches are considered. In the area based estimation, the plot-level forest attributes (such as mean tree height and volume, biomass, etc.) are predicted directly from the ALS data, typically using regression -type methods. In individual tree detection, by contrast, the aim is to derive the plot-level statistics from the individual tree information inferred from the ALS data. In our approach, a set of canopy height models (CHM) are fitted to the ALS data. The animated figure on the left shows an example of the ALS point cloud (the point color illustrates the altitude of the reflection point), and the CHMs (green shapes) corresponding to field measurements (indicated with text ‘measurement’) and ALS data (‘estimate’). In this example case, most of the trees were successfully detected based on the ALS data.
Contact
Past and present collaborators
- M. Maltamo, T. Tokola, P. Packalén, University of Eastern Finland, School of Forest Sciences
- M. Rautiainen, Aalto University, Department of Built Environment