Functional Imaging
Functional imaging of bone
Radiographic images of human femora are common tools for diagnosis in clinics. For example, they are used for evaluating the severity of osteoporosis and osteoarthritis. Our aim is to help diagnosis by building new computational methods that can gather more information from these images.
Measurement of 2D bone mineral density (BMD) with dual-energy X-ray absorptiometry (DXA) imaging of proximal femur is the gold standard method for diagnosis of osteoporosis and for predicting fracture risk. However, the DXA images alone are only a moderate predictor of fractures. We believe that with mechanical modeling we can predict the fractures more accurately since then also the geometry of bone is included in prediction of fracture. Therefore, we developed a method to estimate the 3D shape and internal density of the proximal femur based on one DXA image. Then, this estimated shape can be fed for finite element analysis (FEA) to get a subject specific mechanical model and fracture model. From this model we can calculate the maximum strength that the patient’s proximal femur can bear without fracture.
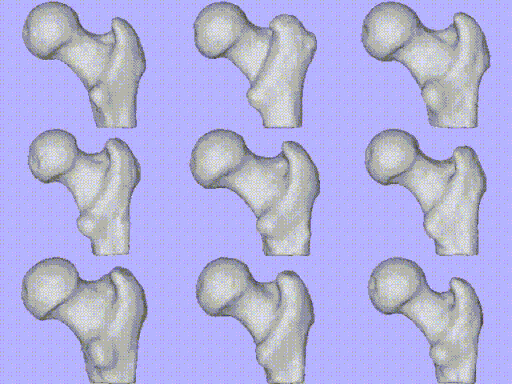
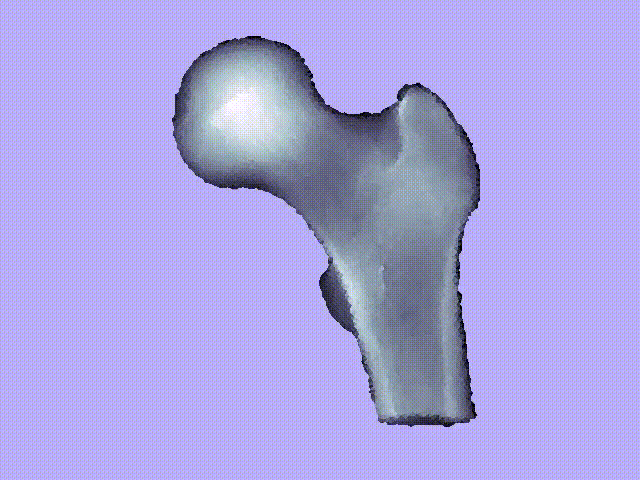
The videos present the estimation of the 3D shape and internal density of a proximal femur based on a 2D DXA image. (a) A template which includes general information about femur shape and density distribution is built from femoral CT images. Then, the shape of this template is fitted to the shape of 2D DXA image and the internal density is matched with the one from the DXA image.
Literature:
Väänänen Sami P., Isaksson Hanna, Julkunen Petro, Sirola Joonas, Kröger Heikki, Jurvelin Jukka S., Assessment of the 3-D shape and mechanics of the proximal femur using a shape template and a bone mineral density image, Biomechanics and modeling in mechanobiology, 4 529—538 (2011)
Väänänen Sami P., Jurvelin Jukka S., and Isaksson Hanna, Estimation of 3-D shape, internal density and mechanics of proximal femur by combining bone mineral density images with shape and density templates, Biomechanics and modeling in mechanobiology 6:791-800 (2012)
A common way to evaluate the geometry of the proximal femur is to take measures from the femoral radiograph, i.e., shape parameters. Then they can be correlated with clinical parameters such as severity of osteoarthritis or osteoporosis to find their relation or to use them as predictor of the clinical parameters. However, each of these shape parameters measure only one local feature of the shape. Therefore, we have built a statistical shape model that can describe the variation in both shape and density of femur in the evaluated population with a few parameters, called modes. The base of the statistical shape model is the principal component analysis. In one application we used the statistical shape model when we created new femur samples from a population. It is possible since the modes describe the variation of the shape and density within the training population (see video). We have also used the statistical shape model for predicting the 3D orientation of the femur in 2D radiographs.
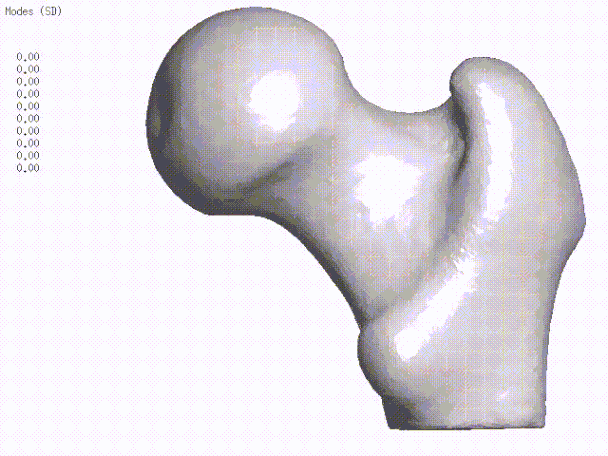
The mode values in the statistical shape model describe the variation of both the shape and internal density in the population’s proximal femora. The value of a mode defines how far the shape is from the average of the population. The unit is standard deviations.
Literature:
Väänänen Sami P., Isaksson Hanna, Waarsing Erwin, Zadpoor Amir Abbas, Jurvelin Jukka S., Weinans Harrie, Estimation of 3D rotation of femur in 2D hip radiographs, Journal of Biomechanics, (2012)
Functional imaging of knee joint
MRI is commonly used for evaluating condition of knee joint. Since MRI offers relatively good contrast between solid and soft tissues, and furthermore, it offers information about compositions and structures in different joint tissues (cartilage, meniscus), it is used for diagnosing degenerative changes in knee joint, such as cartilage and meniscal injuries [1, 2]. However, MRI does not reveal function of knee joint during different physical activity (walking, running). Therefore, different computational models have been developed estimating varying forces and strains in the knee joint.
In the recent studies [3-5], realistic loading input has been implemented into knee joint models. However, these models have lacks in realistic material characterization for different joint tissues. Cartilages of knee joint have been usually considered as linear isotropic material [3-5], while in reality they have highly anisotropic and time-dependent properties due to depth-dependent collagen fibril network, proteoglycan density and fluid fraction [6-8]. Since all knee joint loading forces are transferred through cartilage tissues to bone, effects of previous compositions should be considered as realistically as possible. This is possible by using fibril-reinforced biphasic properties for cartilage tissues.
For simulating realistically effects of different joint loadings in addition to realistic material characterization, varying knee loadings, rotations and translations (movements of femur respect with to tibia) is needed. This data can be obtained by using different gait analysis methods, such as fluoroscopic setup system or markers [9, 10]. When computational model with realistic material characterizations for different joint tissues and realistic data during physical activity is combined, realistic simulations can be done (11-13). This approach can be used for designing and optimizing treatment operations, such as partial meniscectomy or cartilage/menisucs repair. Furthermore, presented tool can be used for estimating development\probability of osteoarthritis in knee joint.
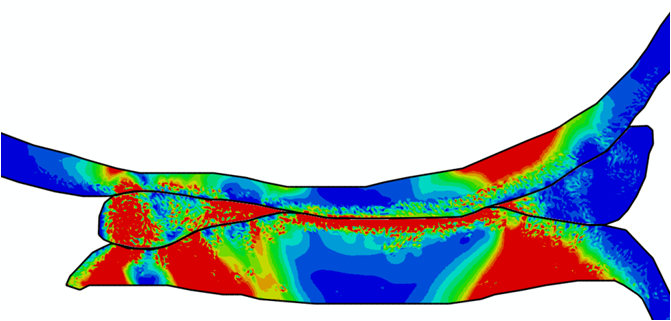
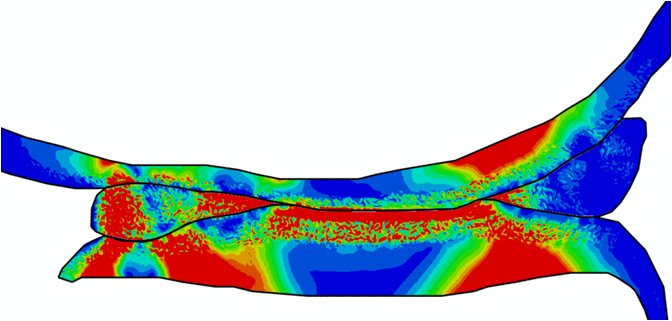
Comparison of stress variations within knee joint during axial impact loading with different collagen fibril architecture in the tibial cartilage: figures show results of different collagen architectures at the center of medial compartment of the knee joint. In the top figure, collagen orientations are based on the T2-mapped MRI data, whereas in the bottom figure, collagen orientations are obtained from the literature.
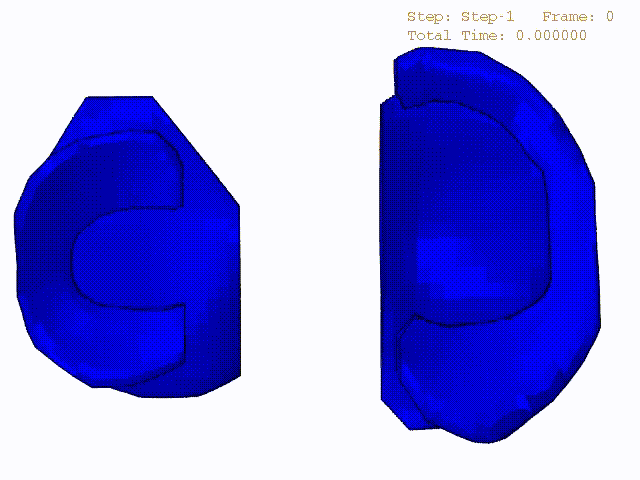
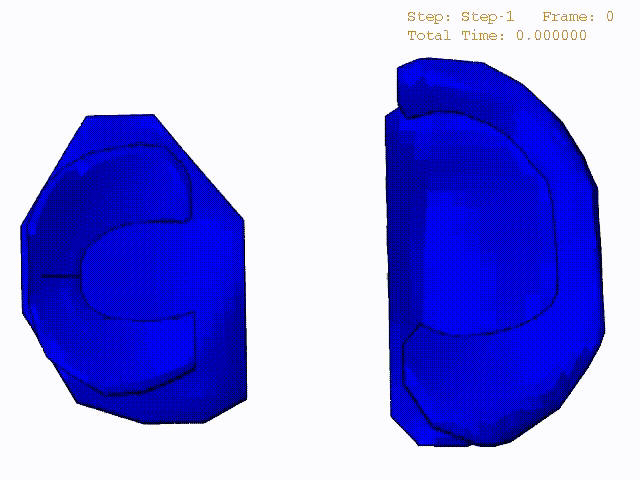
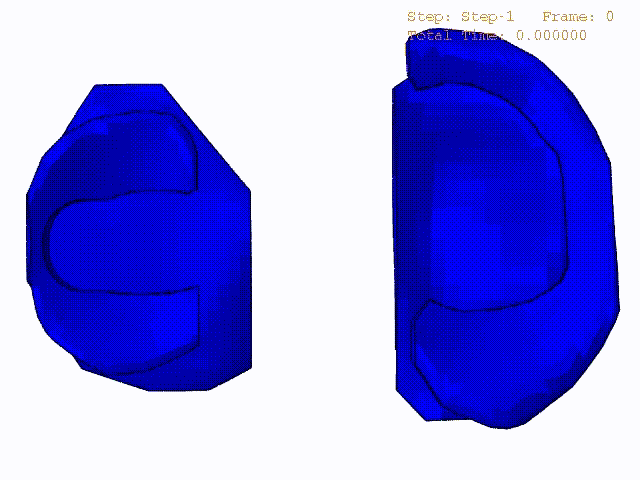
Stress variations in the intact knee joint (top video), in the knee joint with middle radial tear of lateral meniscus (middle video) and in the knee joint with partial lateral meniscectomy during normal walking (bottom video).
References
- Braun, J.J., Gold, G.E., 2012. Diagnosis of osteoarthritis: Imaging. Bone 51:278-88
- Roemer, F.W., Crema, M.D., Trattnig, S., Guermazi, A., 2011. Advances in imaging of osteoarthritis and cartilage. Radiology 260:332-354
- Netravali NA, Koo S, Giori NJ, Andriacchi TP. 2011. The effect of kinematic and kinetic changes on meniscal strains during gait. J Biomech Eng 133:011006.
- Yang NH, Nayeb-Hashemi H, Canavan PK, Vaziri A. 2010. Effect of frontal plane tibiofemoral angle on the stress and strain at the knee cartilage during the stance phase of gait. J Orthop Res 28:1539-1547.
- Saveh AH, Katouzian HR, Chizari M. 2011. Measurement of an intact knee kinematics using gait and fluoroscopic analysis. Knee Surg Sports Traumatol Arthrosc 19:267-272.
- Wilson W., van Donkelaar C.C., van Rietbergen B., Ito K., Huiskes R., 2004. Stresses in the local collagen network of articular cartilage: a poroviscoelastic fibril-reinforced finite element study. J Biomech 37:357-66
- Julkunen P., Kiviranta P., Wilson W., Jurvelin J.S., Korhonen R.K., 2007. Characterization of articular cartilage by combining microscopic analysis with a fibril-reinforced finite-element model. J Biomech 40:1862-70.
- Soltz, M.A., Ateshian, G.A., 1998. Experimental verification and theoretical prediction of cartilage interstitial fluid pressurization at an impermeable contact interface in confined compression. J Biomech 31:927–934 (1998)
- Komistek, R. D., Stiehl, J. B., Dennis, D. A., Paxson, R. D., Soutas-Little, R. W., 1997. Mathematical model of the lower extremity joint reaction forces using Kane’s method of dynamics. J Biomech 31:185-189.
- Kozanek, M., Hosseini, A., Liu, F., Van de Velde, S. K., Gill, T. J., Rubash, H. E., Li, G., 2009. Tibiofemoral kinematics and condylar motion during the stance phase of gait. J Biomech 42:1877-1884.
- Räsänen, L.P., Mononen, M.E., Nieminen, M.T., Lammentausta, E., Jurvelin, J.S., Korhonen, R.K., 2012. Implementation of Subject-Specific Collagen Architecture of Cartilage Into a 2D Computational Model of a Knee Joint—Data From the Osteoarthritis Initiative (OAI). J Orthop Res, 2012. DOI 10.1002/jor.22175
- Mononen, M.E., Mikkola, M.T., Julkunen, P., Ojala, R., Nieminen, M.T., Jurvelin, J.S., Korhonen, R.K., 2011. Effect of superficial collagen patterns and fibrillation of femoral articular cartilage on knee joint mechanics-a 3D finite element analysis. J Biomech 45:579-87
- Mononen, M.E., Julkunen, P., Toyras, J., Jurvelin, J.S., Kiviranta, I., Korhonen, R.K., 2011. Alterations in structure and properties of collagen network of osteoarthritic and repaired cartilage modify knee joint stresses. Biomech Model Mechanobiol 10:357-69.